Truly Tabular RDF
Querying tabular data from Wikidata
A starting point for analyzing the data in a triplestore such as wikidata might be a single item of interest such as the "Game of Thrones" character Jon Snow instance of
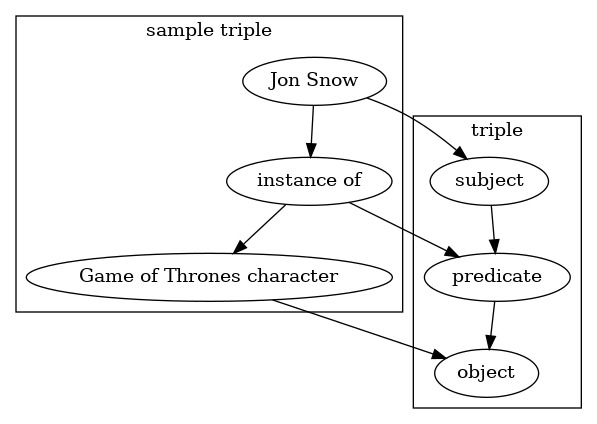
Naive SPARQL Query
- Start with a wikidata item your are intested in e.g. International Semantic Web Conference ISWC 2022
- use the instance of property to find similar items of the same class academic conference
- straight-forward select further properties by adding statements similar to to the WHERE clause.
OPTIONAL { ?conference wdt:P1813 ?short_name }
This naive approach will lead to more results for Step 3 (e.g. 7730) than for step 2 (e.g. 7695) which is a surprise for most novices since this effect would not happen with a similar SQL query
SELECT short_name,country,title from academic_conference
Result of Step #2
# Academic conference wikidata query
# WF 2021-01-30
PREFIX wd: <http://www.wikidata.org/entity/>
PREFIX wdt: <http://www.wikidata.org/prop/direct/>
PREFIX rdfs: <http://www.w3.org/2000/01/rdf-schema#>
SELECT ?conference ?conferenceLabel
WHERE
{
# academic conference series (Q2020153)
?conference wdt:P31 wd:Q2020153.
# label
?conference rdfs:label ?conferenceLabel filter (lang(?conferenceLabel) = "en").
}
conference | conferenceLabel |
---|---|
http://www.wikidata.org/entity/Q75698988 | The 2013 Conference of the North American Chapter of the Association for Computational Linguistics: Human Language Technologies |
http://www.wikidata.org/entity/Q75707991 | Digital Humanities 2020 |
http://www.wikidata.org/entity/Q75709854 | Digital Humanities 2018 |
... |
Result of Step 3
# Academic conference wikidata query
# WF 2021-01-30
PREFIX wd: <http://www.wikidata.org/entity/>
PREFIX wdt: <http://www.wikidata.org/prop/direct/>
PREFIX rdfs: <http://www.w3.org/2000/01/rdf-schema#>
SELECT
?conference ?conferenceLabel
?short_name
?country
?title
WHERE
{
# academic conference series (Q2020153)
?conference wdt:P31 wd:Q2020153.
# label
?conference rdfs:label ?conferenceLabel filter (lang(?conferenceLabel) = "en").
# short name
OPTIONAL { ?conference wdt:P1813 ?short_name }
# country
OPTIONAL { ?conference wdt:P17 ?country }
# title
OPTIONAL { ?conference wdt:P1476 ?title }
}
More elaborate example: novel series
- start with Lord of the Rings
- find similar Novel Series
Naive SPARQL Query
# truly tabular query for
# Q1667921:novel series
# generated by trulytabular.py on 2022-07-27T17:33:43.681991
PREFIX wd: <http://www.wikidata.org/entity/>
PREFIX wdt: <http://www.wikidata.org/prop/direct/>
PREFIX rdfs: <http://www.w3.org/2000/01/rdf-schema#>
SELECT ?novel_series ?novel_seriesLabel
?instance_of
?language_of_work_or_name
?genre
?author
?country_of_origin
?has_part_s_
?publication_date
?Freebase_ID
?ISFDB_series_ID
?title
?Google_Knowledge_Graph_ID
WHERE {
# instanceof Q1667921:novel series
?novel_series wdt:P31 wd:Q1667921.
# label
?novel_series rdfs:label ?novel_seriesLabel
FILTER (LANG(?novel_seriesLabel) = "en").
# instance of (P31)
OPTIONAL { ?novel_series wdt:P31 ?instance_of. }
# language of work or name (P407)
OPTIONAL { ?novel_series wdt:P407 ?language_of_work_or_name. }
# genre (P136)
OPTIONAL { ?novel_series wdt:P136 ?genre. }
# author (P50)
OPTIONAL { ?novel_series wdt:P50 ?author. }
# country of origin (P495)
OPTIONAL { ?novel_series wdt:P495 ?country_of_origin. }
# has part(s) (P527)
OPTIONAL { ?novel_series wdt:P527 ?has_part_s_. }
# publication date (P577)
OPTIONAL { ?novel_series wdt:P577 ?publication_date. }
# Freebase ID (P646)
OPTIONAL { ?novel_series wdt:P646 ?Freebase_ID. }
# ISFDB series ID (P1235)
OPTIONAL { ?novel_series wdt:P1235 ?ISFDB_series_ID. }
# title (P1476)
OPTIONAL { ?novel_series wdt:P1476 ?title. }
# Google Knowledge Graph ID (P2671)
OPTIONAL { ?novel_series wdt:P2671 ?Google_Knowledge_Graph_ID. }
}
Aggregate SPARQL Query with SAMPLE
# truly tabular query for
# Q1667921:novel series
# generated by trulytabular.py on 2022-07-27T17:33:43.681991
PREFIX wd: <http://www.wikidata.org/entity/>
PREFIX wdt: <http://www.wikidata.org/prop/direct/>
PREFIX rdfs: <http://www.w3.org/2000/01/rdf-schema#>
SELECT DISTINCT ?novel_series ?novel_seriesLabel
(SAMPLE (?instance_of) AS ?instance_of )
(SAMPLE (?language_of_work_or_name) AS ?language_of_work_or_name)
(SAMPLE (?genre) AS ?genre)
(SAMPLE (?author) AS ?author)
(SAMPLE (?country_of_origin) AS ?country_of_origin)
(SAMPLE (?has_part_s_) AS ?has_part_s_)
(SAMPLE (?publication_date) AS ?publication_date)
(SAMPLE (?Freebase_ID) AS ?Freebase_ID)
(SAMPLE (?ISFDB_series_ID) AS ?ISFDB_series_ID)
(SAMPLE (?title) AS ?title )
(SAMPLE (?Google_Knowledge_Graph_ID) AS ?Google_Knowledge_Graph_ID)
WHERE {
# instanceof Q1667921:novel series
?novel_series wdt:P31 wd:Q1667921.
# label
?novel_series rdfs:label ?novel_seriesLabel
FILTER (LANG(?novel_seriesLabel) = "en").
# instance of (P31)
OPTIONAL { ?novel_series wdt:P31 ?instance_of. }
# language of work or name (P407)
OPTIONAL { ?novel_series wdt:P407 ?language_of_work_or_name. }
# genre (P136)
OPTIONAL { ?novel_series wdt:P136 ?genre. }
# author (P50)
OPTIONAL { ?novel_series wdt:P50 ?author. }
# country of origin (P495)
OPTIONAL { ?novel_series wdt:P495 ?country_of_origin. }
# has part(s) (P527)
OPTIONAL { ?novel_series wdt:P527 ?has_part_s_. }
# publication date (P577)
OPTIONAL { ?novel_series wdt:P577 ?publication_date. }
# Freebase ID (P646)
OPTIONAL { ?novel_series wdt:P646 ?Freebase_ID. }
# ISFDB series ID (P1235)
OPTIONAL { ?novel_series wdt:P1235 ?ISFDB_series_ID. }
# title (P1476)
OPTIONAL { ?novel_series wdt:P1476 ?title. }
# Google Knowledge Graph ID (P2671)
OPTIONAL { ?novel_series wdt:P2671 ?Google_Knowledge_Graph_ID. }
} GROUP BY ?novel_series ?novel_seriesLabe
How tabular are the Academic Conference entries in wikidata?
Result as of 2022-03
property | total | f1 | total% | non tabular | non tabular% | f2 | f3 | f14 | f4 | f7 | f5 | f9 |
---|---|---|---|---|---|---|---|---|---|---|---|---|
∑ | 7518 | |||||||||||
short name | 6750 | 6741 | 89.8 | 9 | 0.1 | 9 | ||||||
country | 7077 | 7077 | 94.1 | 0 | 0 | |||||||
title | 6718 | 6700 | 89.4 | 18 | 0.3 | 10 | 8 | |||||
part of the series | 7139 | 7120 | 95 | 19 | 0.3 | 15 | 4 | |||||
VIAF ID | 2096 | 2092 | 27.9 | 4 | 0.2 | 3 | 1 | |||||
GND ID | 3049 | 3043 | 40.6 | 6 | 0.2 | 4 | 2 | |||||
location | 7209 | 7180 | 95.9 | 29 | 0.4 | 24 | 4 | 1 | ||||
start time | 6916 | 6914 | 92 | 2 | 0 | 2 | ||||||
end time | 6912 | 6909 | 91.9 | 3 | 0 | 3 | ||||||
official website | 596 | 586 | 7.9 | 10 | 1.7 | 9 | 1 | |||||
main subject | 1882 | 1722 | 25 | 160 | 8.5 | 131 | 23 | 2 | 2 | 1 | 1 | |
described at URL | 6512 | 6510 | 86.6 | 2 | 0 | 1 | 1 | |||||
language used | 87 | 84 | 1.2 | 3 | 3.4 | 3 | ||||||
is proceedings from | 921 | 901 | 12.3 | 20 | 2.2 | 16 | 3 | 1 | ||||
WikiCFP event ID | 98 | 98 | 1.3 | 0 | 0 |